Data Governance & Quality
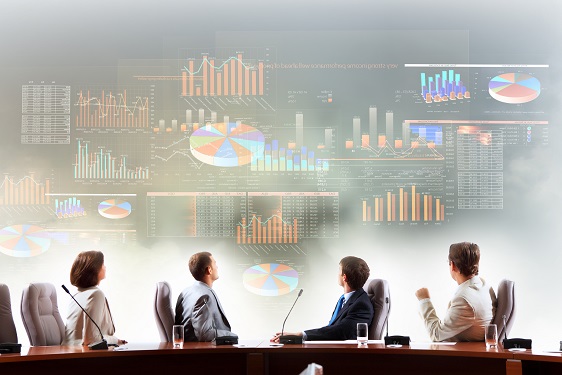
The value of the information asset is realized when information is used to improve a process or enable fact-based decision making. However, many organizations fail to put good controls around data to ensure accuracy, understanding and transparency. More and more data is shared with other areas within an organization as well as with external consumers of information. The same data may be interpreted to any number of meanings without appropriate context and data quality controls in place. According to Gartner Research published in October 2011, data quality affects overall labor productivity by as much as a 20%. Poor data quality is a primary reason for 40% of all business initiatives failing to achieve their targeted benefits. Gartner continued to observe a high correlation between significant data quality issues and degraded productivity of key resources.
Other organizations rely solely on technology to improve data quality at points in the information lifecycle as opposed to tracing the lineage of a data defect to improve quality at the source. The answer is a mix of the two - functional responsibility and governance for data by the information workers and enabling technology to apply governance on a large scale in real time.
The importance of data quality is often underestimated in data migration and integration solutions. Even after years of project failures and mounting evidence, data quality is still the number one cause of data integration project failure or delay. A robust data quality program as part of an overall data governance program is the bedrock of any data migration or integration project.
Any information management program must use a transparent method and guiding principles to realize maximum value of the investment in information as an enterprise asset. There are a few guiding principles that we use when standing up a data governance group. These typically form the basis of a program charter document. From this base set we incorporate any burning issues and begin formulation of program performance measures.
- Governance for the data management environment is performed by cross-functional teams, who prioritize, monitor initiatives and needs, and speedily resolve major conflicts.
- Data quality and lineage is monitored, based on business value and accountability, for information throughout its lifecycle.
- The information architecture is incrementally deployed to accommodate evolving business needs, tools, and technologies and is consistent with industry models.
- The information management program establishes an information catalog of met information needs to support reuse of existing assets.
- The information management environment accommodates rapid business change and enables enhanced use of information.
- Information management capabilities, including their applicability and limitations, are effectively communicated.
The data governance functional model clearly outlines responsibility and accountability for maintaining the value of the organizational information asset. The complexity of the model varies with the complexity and size of the organization. The functions in the model are required no matter the organizational reporting structure.
Data stewardship is at the core of data governance. It is through this group of business data subject matter experts that an organization’s true data potential is realized – or not. No matter the executive support or funding, a well-organized, trained and managed stewardship organization is vital to the success of any data governance initiative. Each major business function should have stewardship. Data stewards must reside in the business and never in IT. They must be visible, respected and influential.
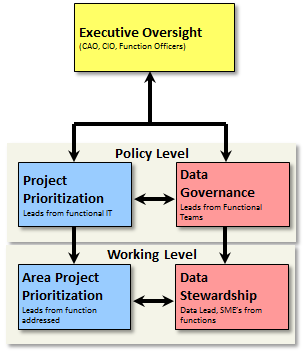
Data stewards must have insight and vision to understand:
- The importance of data quality
- Overall business objectives and context
- Overall impact of quality issues – strategically and tactically
A key facet of any program is the ability to measure performance and evolution. IUI uses the Data Maturity Model depicted below as a means to measure baseline and plan progress. It is important to note that the Data Maturity Model is not a scoring mechanism. It is a communication and planning tool. Organizations are successful at the lower levels of maturity – it just usually takes more effort to be successful.
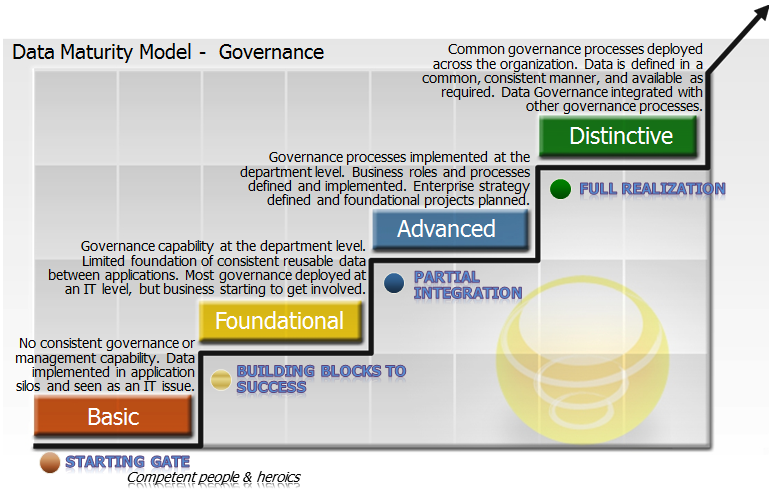
Case Studies
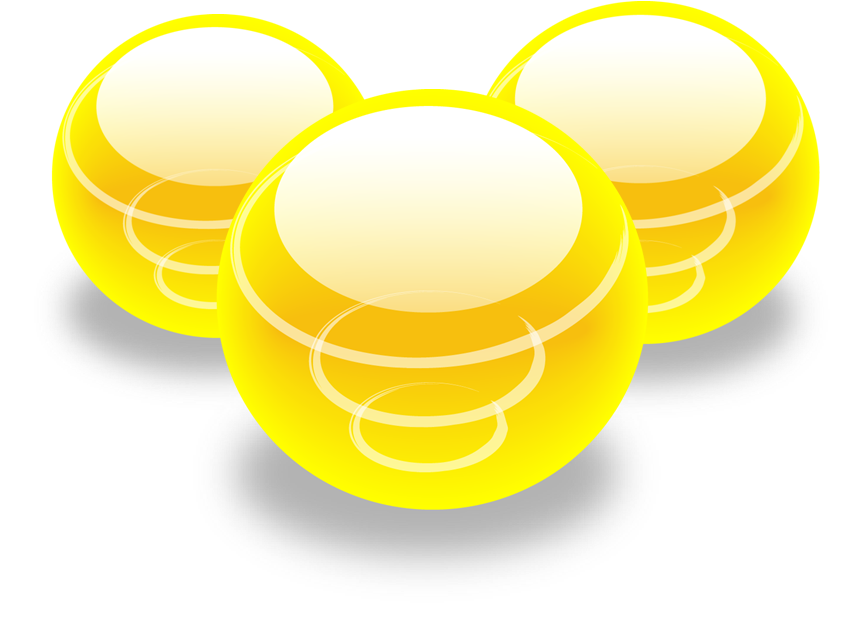
IUI enables fact-based decision making through our holistic focus on Enterprise Information Management. We collect data. We integrate it. We put process around the data.